Trusted by leading companies:
Problem
Sure-fire Security That
SaaS Can’t Offer.
Old-school SaaS-based API security solutions can no longer
keep up with the rapidly evolving, AI-led tech landscape.
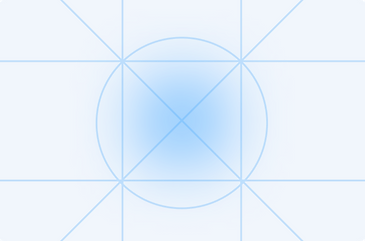
Data privacy at risk
SaaS solutions can store sensitive data
outside your data ecosystem, putting
compliance and sovereignty on the line.

Dependence on third parties
When you rely on third parties for updates
and threat response, you lose control over
your own security.
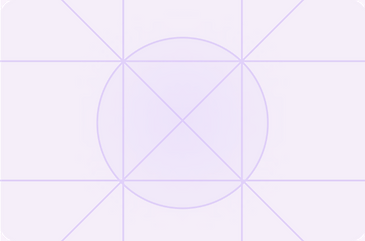
Shared threats and vulnerabilities
Your APIs are only as secure as your SaaS
provider. Their mistakes or vulnerabilities
become your problem.

Solution
The Air Gap Edge with
Ammune.ai
Move over SaaS-based solutions. Our air-gapped autonomous
AI model keeps your data within your network only.
Autonomous Protection
Fully automated enforcement that
generates protection policies from the
very first anomaly.
Platform Agnostic Scalability
Works everywhere. On-premises,
in the cloud, on containers, and on
DPUs.
Air-Gapped Security
No leaks. No risks. Keeps your data
completely isolated and secure.
Next-Generation AI
Autonomous AI that adapts, learns, and protects without manual intervention.
So you can maximize the potential of
APIs and experience:
High-security Industry Focus
To meet the rigorous demands of finance,
healthcare, government & defence industries.
Unique Isolated Solution
That can operate in offline or semi-isolated
infrastructures.
Compliance & Trust
With regulatory requirements like
GDPR and HIPAA
Explore Our Airgap Solution
Experience revolutionary AI-led API security tailored to your specific
organizational requirements through Ammune.ai’s platforms:
API Discovery
Know Every API.
No Exceptions.
API WAF
Stop Content-based Attacks.
Without delays.
API BOT
We Crush Bots.
Period.
API-DDoS
DDoS?
Not on Our Watch.
API BL
Fraud Stops Here.
Awards
Most Follow Standards. We Set Them.
Ammune.ai certified by industry- leading third-party standards
Product Leadership Award 2020

10 Most Trusted Cybersecurity Companies, 2021

Testimonials
Real Stories. Real Security
Ammune (server side) bot protection achieves superior results
for bot detection (client side), Without any need for team
intervention. It is a close to Zero-Touch solution that saves us
a lot of effort. Ammune is fully integrated with the F5 LB.
Ammune protection automation and precision as well as the
API visibility are critical to our digital business.
Ammune self-managed API security solution fits our needs
as we have a small team and automation is a must.
Ammune applicative DDOS protection detects
under the radar attacks that pass through Gov.il
entire protection pipeline. It is a major reason to
our decision to add ammune solution to the pipe